Blog
Check out the latest blog articles from Sesame Software.
To always be in the know, subscribe below!
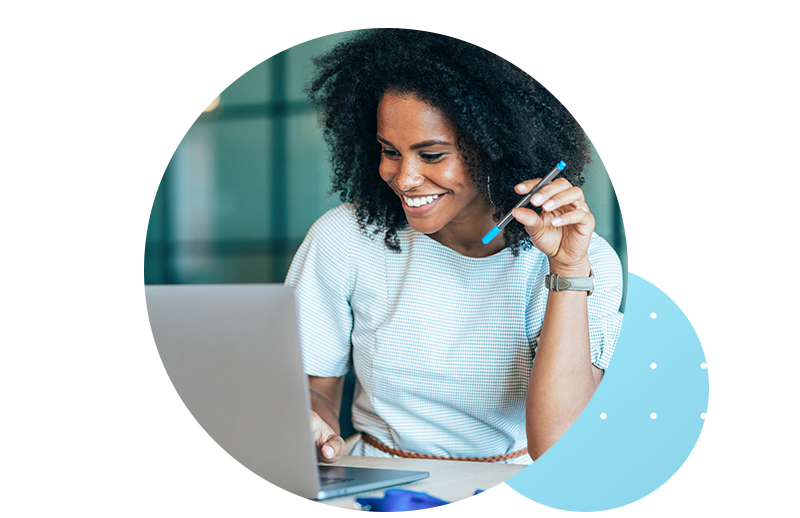
How to Prevent Deadlocks and Data Failures During Large-Scale Transfers
At Sesame Software, preventing deadlocks and data failures isn’t just theory - it’s a practice we’ve refined for over three decades. Our platform was built specifically to tackle the complex...
Migrating from Pardot? Here’s How to Keep Your Data Intact
Switching from Pardot to a new marketing platform can feel overwhelming - especially when it comes to your data. Pardot (now known as Marketing Cloud Account Engagement) is designed for B2B...
How to Back Up Salesforce Data Using Sesame Software
Backing up your Salesforce data isn’t just a precaution—it’s a core part of any resilient data strategy. Whether you're preparing for accidental deletions, data corruption, or compliance audits,...
What Rising Platform Prices Mean for Your Data Strategy
Over the past year, we’ve seen a wave of price increases across major platforms in the data ecosystem - especially when it comes to backup tools and cloud storage. Whether it’s a bump in licensing...
How Colleges Use Sesame Software to Power Their Websites and Salesforce Integration
Higher education institutions rely on fast, reliable access to data to support every stage of the student lifecycle—from recruitment and enrollment to engagement and advancement. Whether it’s...
Understanding Role-Based Access Control (RBAC) in Data Management
Managing data access effectively is critical for security and operational efficiency, and Role-Based Access Control (RBAC) provides a structured way to ensure users only have access to the data they...
Columnar Database vs. Relational Database: How Sesame Software Handles Both Efficiently
Data storage and processing have evolved significantly over the years, but businesses today still face the challenge of choosing the right type of database for their needs. Two of the most common...
World Backup Day
In today's digital age, data has become an integral part of our lives, and it's essential to keep it safe and secure from any potential threats. World Backup Day is celebrated every year on March...
Data Replication Types and Their Benefits: Finding the Right Solution with Sesame Software
Accurate, up-to-date information is essential for businesses to make confident decisions. Data replication — the process of copying and synchronizing data across systems — plays a key role in...
The Global Shift Toward Standardized Digital Bookkeeping
In today’s highly interconnected world, governments are tightening regulations on bookkeeping and financial data storage. Standardized digital bookkeeping systems are being mandated to ensure tax...
Unlocking Business Potential Through Data Consulting Projects
In a world where data drives decision-making, businesses often find themselves at crossroads—struggling to keep up with scaling systems or stuck with outdated processes that hinder growth. That’s...
How to Unlock More Value from Your Data to Start Q2 and Win Big
Is your data strategy a winning hand or a risky gamble? Every business knows that data is invaluable—but what if optimizing your data could actually pay off? Every day, your business generates...
Unlocking the Power of Business Intelligence for Small Businesses
No matter your line of business, data is a key driver of success. While large enterprises have long leveraged Business Intelligence (BI) tools to gain insights and streamline operations, small...
Preventing Unauthorized Access: Best Practices for Data Security
In the ever-evolving landscape of cyber threats, unauthorized access remains a significant concern for organizations of all sizes. As a leading provider of data management solutions, Sesame Software...
Your Data Is Yours: How Sesame Software Reduces Risk by Not Retaining It
In today’s digital age, data is invaluable—but it’s also one of the biggest liabilities a company can hold. Headlines are filled with stories of data breaches exposing sensitive information and...